How AI-Generated Text Automation Can Benefit Freelance Writers with Automated AI for Data-Driven Content Creation
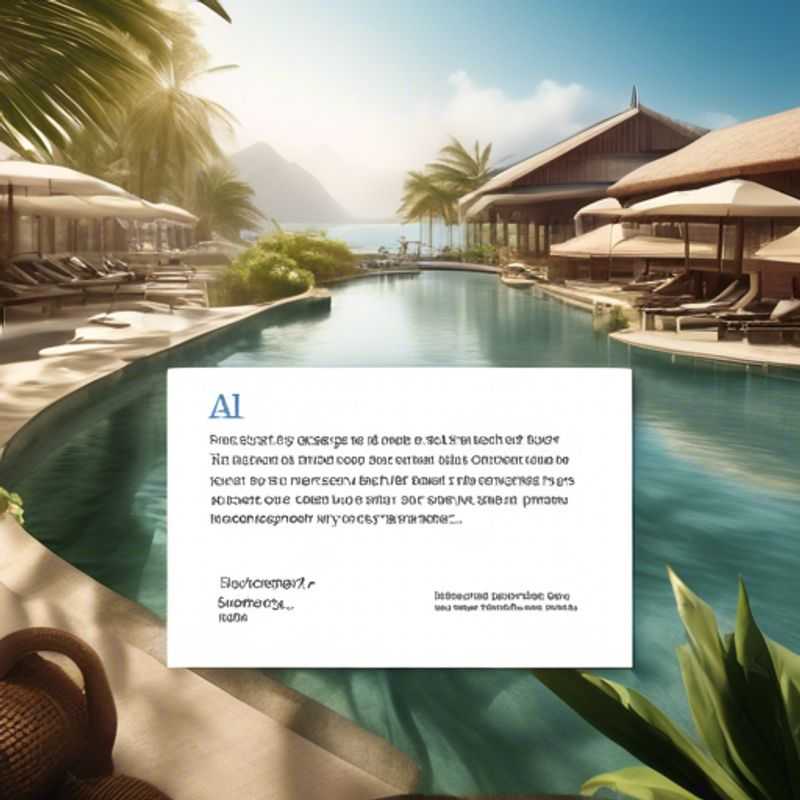
How AI-Generated Text Automation Can Supercharge Your Freelance Writing: A Data-Driven Approach
The world of freelance writing is rapidly evolving, and AI-powered text automation is at the forefront of this change. By leveraging the power of AI, freelance writers can unlock new levels of efficiency and creativity, transforming their workflow and ultimately, their success.
At the heart of this transformation lies the ability to utilize pre-trained language models for generating high-quality text. These models, trained on vast amounts of data, can understand the nuances of language and generate text that is both compelling and informative. This opens up a world of possibilities for freelance writers, from crafting engaging blog posts to generating persuasive marketing copy.
To further enhance this process, techniques like transfer learning can be implemented to fine-tune models for specific use cases. By leveraging existing knowledge from a general language model and adapting it to a particular niche, writers can ensure their AI-generated content is tailored to their target audience and resonates with their specific needs.
The success of AI-powered text automation hinges on the quality of data fed to the models. Robust data preprocessing pipelines are essential to ensure model inputs are clean, consistent, and free from errors. This meticulous preparation ensures the models receive accurate information, leading to more reliable and accurate outputs.
The art of generating fluent and coherent text lies in the decoding strategies employed. Experimentation with different decoding techniques is key to optimizing for natural language flow and ensuring the generated content reads smoothly and engagingly.
The power of human feedback cannot be underestimated. By incorporating human input and iteratively refining the generated text, writers can continuously improve model performance. This iterative approach ensures the AI-generated content aligns with the writer's vision and meets the highest standards of quality.
As with any powerful tool, it's crucial to implement safeguards to prevent the generation of harmful or biased content. By integrating ethical considerations into the development and deployment of AI-powered text automation, writers can ensure their creations are responsible and contribute positively to the world.
The journey of AI-powered text automation is ongoing. Continuously monitoring model outputs and refining prompts are essential to maintain quality over time. By embracing this dynamic process and staying informed about the latest advancements, freelance writers can harness the full potential of this technology and elevate their writing to new heights.
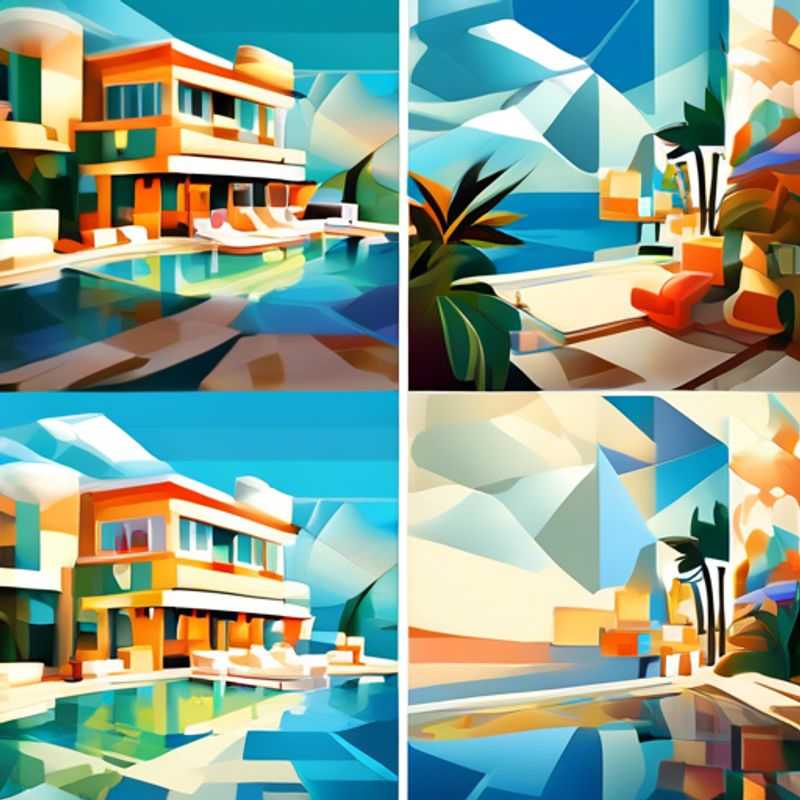
Unlocking Creativity: Using Pre-trained Language Models for Text Generation
Pre-trained language models are powerful tools for generating high-quality text. They are trained on massive datasets of text, allowing them to learn the nuances of language and produce coherent, engaging content. This technology has revolutionized the way we create content, offering numerous benefits for individuals and businesses.
One of the key advantages of using pre-trained language models is their ability to generate text that is both creative and factual. These models can understand context, generate different writing styles, and even come up with original ideas. This makes them incredibly useful for tasks like writing blog posts, articles, social media content, and even creative fiction.
Moreover, pre-trained language models can significantly improve workflow efficiency. They can automate repetitive tasks like generating product descriptions, summarizing lengthy documents, or translating text. This frees up time for you to focus on more strategic and creative aspects of your work.
It's important to note that pre-trained language models are not a substitute for human writers. They are powerful tools that can enhance your content creation process, but they still require human oversight and guidance. It's crucial to proofread and edit the generated text to ensure accuracy, clarity, and brand consistency.
As for costs, accessing and utilizing pre-trained language models can vary depending on the platform and model you choose. Some platforms offer free tiers, while others require paid subscriptions or API usage fees. It's essential to consider your specific needs and budget when choosing a pre-trained language model.
In conclusion, pre-trained language models offer a game-changing solution for generating high-quality text. They are versatile, efficient, and capable of producing both creative and factual content. While human oversight is still required, these models can significantly enhance workflow efficiency and elevate the quality of your writing.
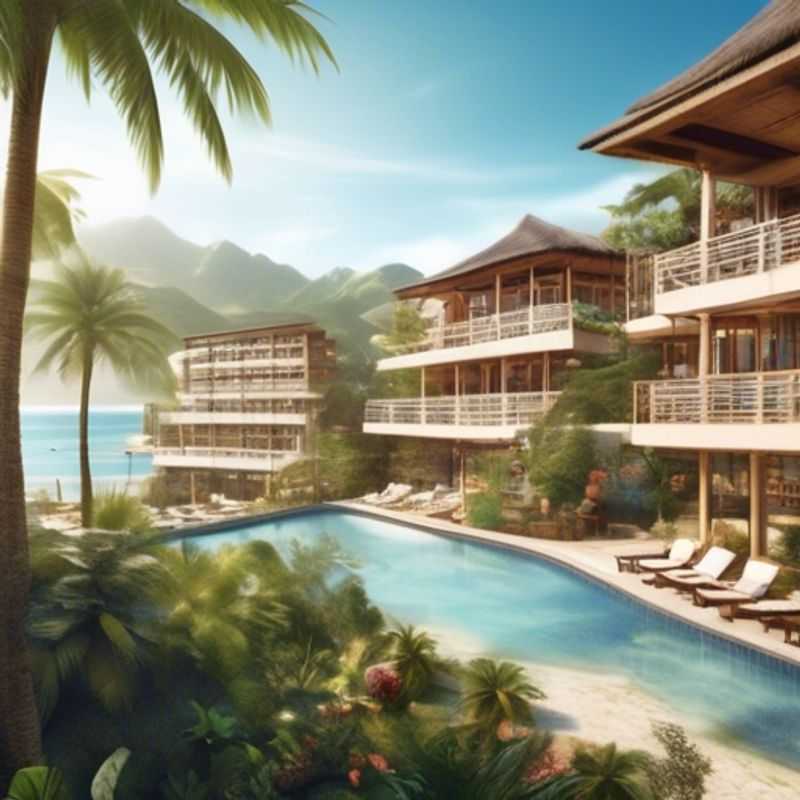
Supercharge Your Models: Transfer Learning for Tailored Performance
Transfer learning is a powerful technique for fine-tuning pre-trained models to specific use cases. It allows you to leverage the knowledge and patterns learned from a massive dataset, saving you time and resources in training your own model from scratch. Imagine you have a pre-trained model that understands the English language; you can then use transfer learning to adapt it for tasks like sentiment analysis or text summarization for your specific domain, like customer reviews or legal documents. This means you don't have to start from scratch and train the model on a huge amount of data just for that specific use case.
The process involves taking a pre-trained model, typically a neural network, and modifying its final layers to adapt it to your specific task. This is done by training the model further on a smaller, domain-specific dataset. For instance, if you're working with medical text, you'd use a medical dataset to fine-tune the model. The pre-trained model acts as a strong foundation, and the fine-tuning process adds the necessary domain-specific knowledge.
Here are the key benefits of using transfer learning:
1. Faster Training: Transfer learning significantly reduces training time compared to starting from scratch. You leverage the pre-trained model's knowledge, making your model learn much faster.
2. Improved Performance: By fine-tuning a powerful pre-trained model, you often achieve better performance on your specific task. The model has already learned general patterns and features, allowing it to perform well even with limited domain-specific data.
3. Less Data Required: You need less data to fine-tune the model compared to training a model from scratch. This is particularly beneficial for domains where large amounts of data are difficult or expensive to obtain.
Transfer learning is a popular technique in various fields, including natural language processing, computer vision, and robotics. It can be applied to a wide range of tasks, such as image classification, object detection, machine translation, and sentiment analysis. To implement transfer learning effectively, you need a good understanding of deep learning and the specific task you're trying to solve. There are various pre-trained models available online, and choosing the right one for your use case is crucial. Remember, the success of transfer learning depends on the quality of the pre-trained model and the relevance of the fine-tuning dataset.
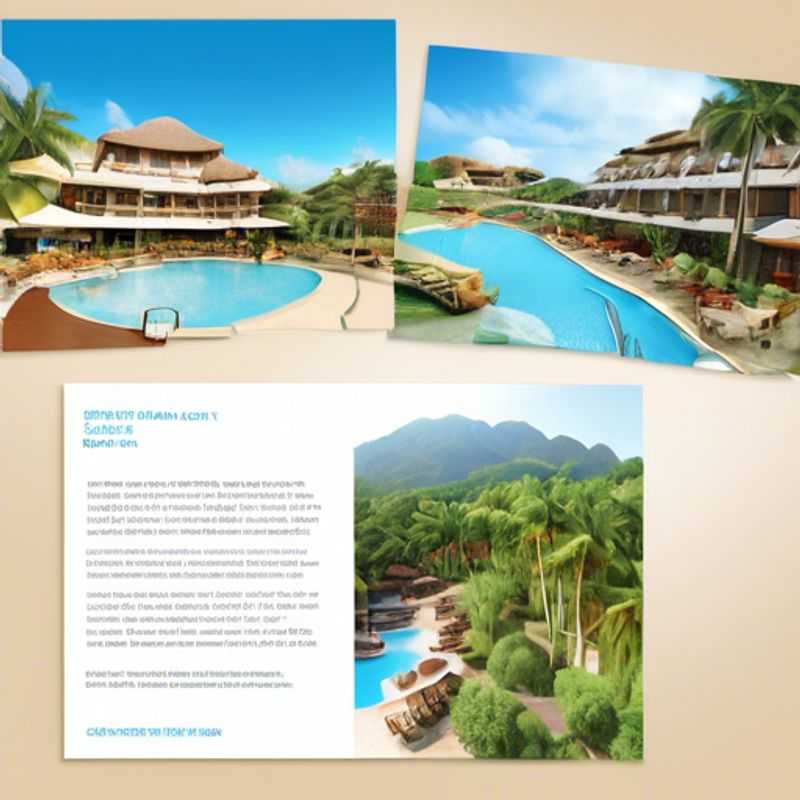
Building Robust Data Preprocessing Pipelines: Clean and Consistent Inputs for Your Models
Data preprocessing is a critical step in building robust machine learning models. It involves transforming raw data into a format suitable for model training. This ensures your model receives clean, consistent inputs, leading to better performance and accuracy.
Think of it as preparing your ingredients before cooking: you wouldn't throw raw vegetables into a pan without cleaning and chopping them first! The same logic applies to machine learning models.
Here's a simplified overview of key data preprocessing techniques:
1. Handling Missing Values: Imagine a recipe missing an ingredient! Missing values in data can cause errors in your model. Techniques include:
• Deletion: Removing rows or columns with missing values.
• Imputation: Replacing missing values with estimates (e.g., using mean, median, or predictive models).
2. Data Cleaning: Removing inconsistencies and errors in your data.
• Outlier Detection: Identifying values that are significantly different from the rest.
• Duplicate Removal: Removing duplicate entries.
3. Data Transformation: Converting data into a format suitable for your model.
• Scaling: Rescaling values to a common range (e.g., between 0 and 1).
• Encoding: Converting categorical features (e.g., colors, labels) into numerical values.
Building a Pipeline:
Instead of applying each preprocessing step individually, consider creating a data preprocessing pipeline. This automates the entire process and ensures consistency.
Key Benefits:
• Improved model accuracy and performance.
• Reduced errors and inconsistencies.
• Enhanced model interpretability.
• Streamlined workflow and efficiency.
Remember, preprocessing is a crucial step in building robust machine learning models. Invest time in understanding and implementing appropriate techniques to ensure your models receive the best possible data inputs.
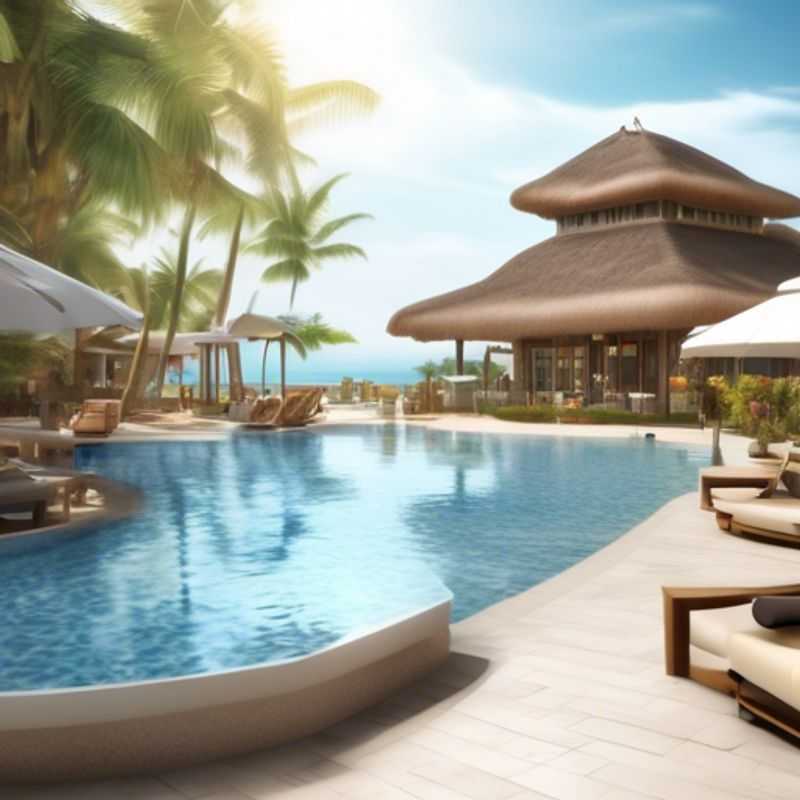
Decoding Strategies: Optimizing for Coherence and Fluency
Decoding strategies in natural language processing are essential for achieving coherence and fluency in machine-generated text. By experimenting with different decoding approaches, you can optimize your model's output to be more human-like and engaging.
Beam Search is a popular decoding method that explores multiple candidate sequences at each step, selecting the most likely one based on a probability score. This approach helps to avoid getting stuck in local optima and improves the overall quality of the generated text. Another technique is Top-k Sampling, where you sample the next word from the top k most probable words in the vocabulary. This method introduces diversity and randomness, which can lead to more creative and surprising outputs.
Nucleus Sampling, a variation of Top-k Sampling, further refines the selection process by filtering out the least probable words based on a threshold parameter. This method helps to control the randomness while still allowing for exploration of diverse options. Lastly, Temperature Scaling adjusts the probabilities of the output distribution, making it more or less predictable. By manipulating the temperature parameter, you can fine-tune the balance between coherence and creativity.
Experimenting with these decoding strategies and fine-tuning their parameters can significantly impact the quality and fluency of your model's outputs. You can adjust them based on your specific task and desired style, aiming for a balance between coherence and creativity.
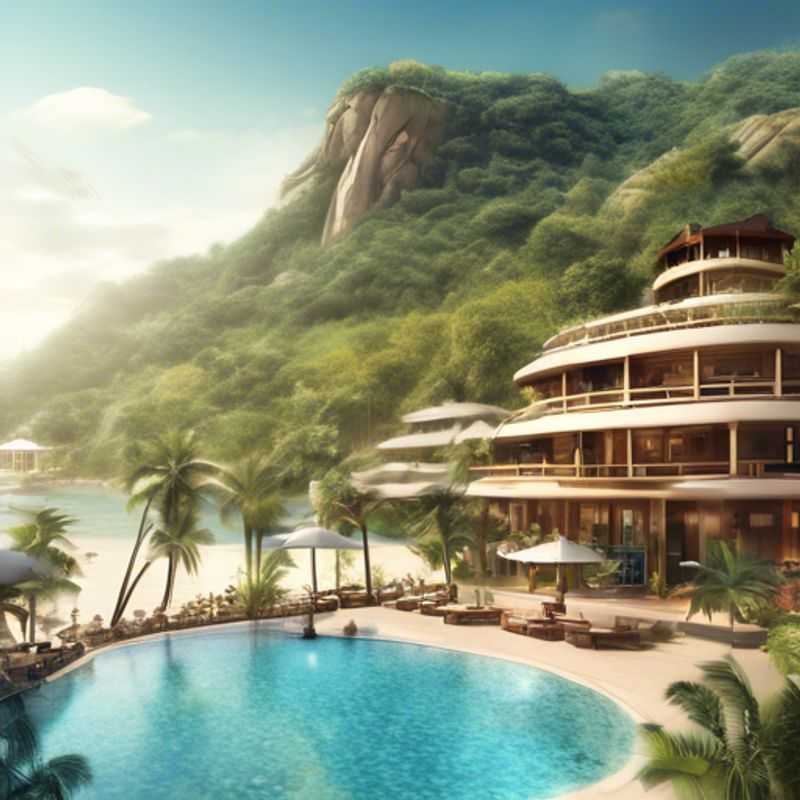
Human Feedback and Iterative Refinement: The Key to Unlocking Model Potential
Leveraging human feedback is a powerful technique for enhancing machine learning model performance. This involves collecting user input, analyzing it to identify areas for improvement, and then iteratively refining the model based on these insights. This approach, known as human-in-the-loop learning, is essential for achieving high-quality results.
Here are some key steps in leveraging human feedback:
1. Identify areas for improvement: Focus on the most critical aspects of your model's performance, such as accuracy, bias, or user experience.
2. Collect feedback: Design methods for gathering user feedback, whether through surveys, A/B testing, or directly incorporating users into the model development process.
3. Analyze feedback: Analyze the collected feedback to understand patterns, trends, and specific areas needing improvement.
4. Refine the model: Use the insights gained from feedback to adjust model parameters, update training data, or improve the model's architecture.
5. Iterate and evaluate: Repeat this process of gathering feedback, analyzing it, and refining the model. This continuous iteration helps to drive ongoing performance improvements.
Incorporating human feedback into your machine learning workflow can significantly improve model accuracy, reduce bias, and enhance user satisfaction. While this process may involve some initial effort, the benefits in terms of improved model performance and user experience often outweigh the costs. Remember, continuous improvement is key to achieving the best possible results with your machine learning models.
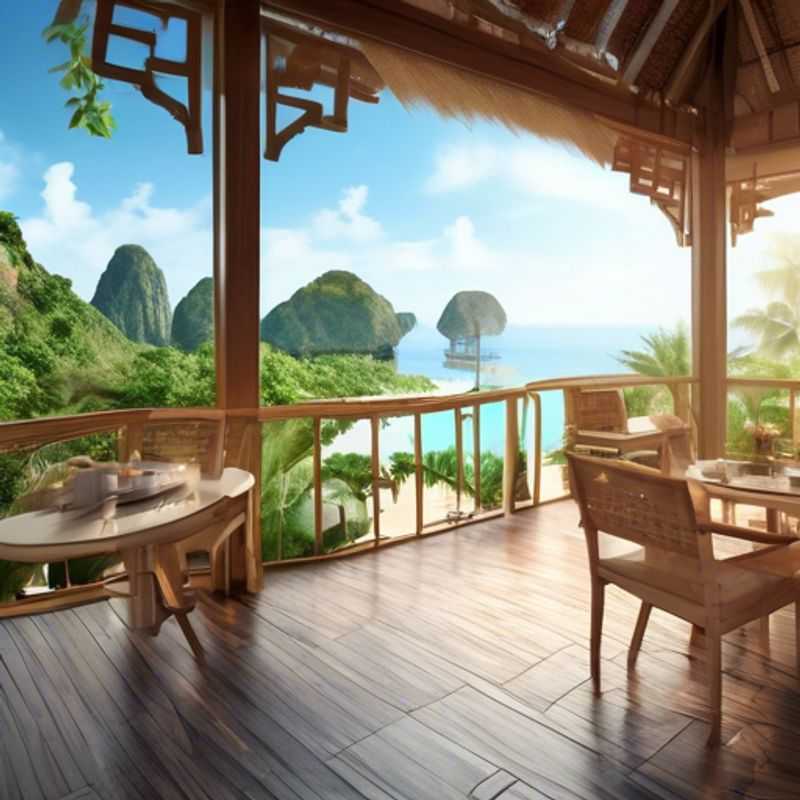
Building Safeguards: Preventing Harmful and Biased Content Generation
Implementing safeguards to prevent harmful or biased content generation in AI systems is crucial. Here's a concise guide to key strategies:
Data Quality & Diversity: Train AI models on diverse, balanced, and high-quality datasets. This helps reduce biases ingrained in the training data. Ensure data is representative of real-world demographics and perspectives.
Human Oversight & Feedback: Incorporate human review processes to monitor generated content. This allows for identification and correction of harmful or biased outputs, ensuring alignment with ethical guidelines.
Content Moderation Systems: Utilize sophisticated algorithms and human reviewers to identify and remove harmful content. These systems can be tailored to detect specific types of bias, hate speech, or misinformation.
Transparency & Explainability: Develop AI models that provide insights into their decision-making processes. This transparency allows for better understanding of potential biases and facilitates accountability.
Regular Updates & Monitoring: Continuously monitor and evaluate the performance of AI models to identify and address emerging biases or harmful content generation patterns. Update models and safeguards as needed.
Ethical Considerations & Guidelines: Establish clear ethical guidelines for AI development and deployment. These guidelines should address fairness, accountability, and responsible use of AI technology.
Remember that building safeguards is an ongoing process. It requires a multi-faceted approach that involves careful data selection, human oversight, sophisticated algorithms, and continuous monitoring. It's essential to invest in these measures to ensure the safe and responsible development and deployment of AI systems.
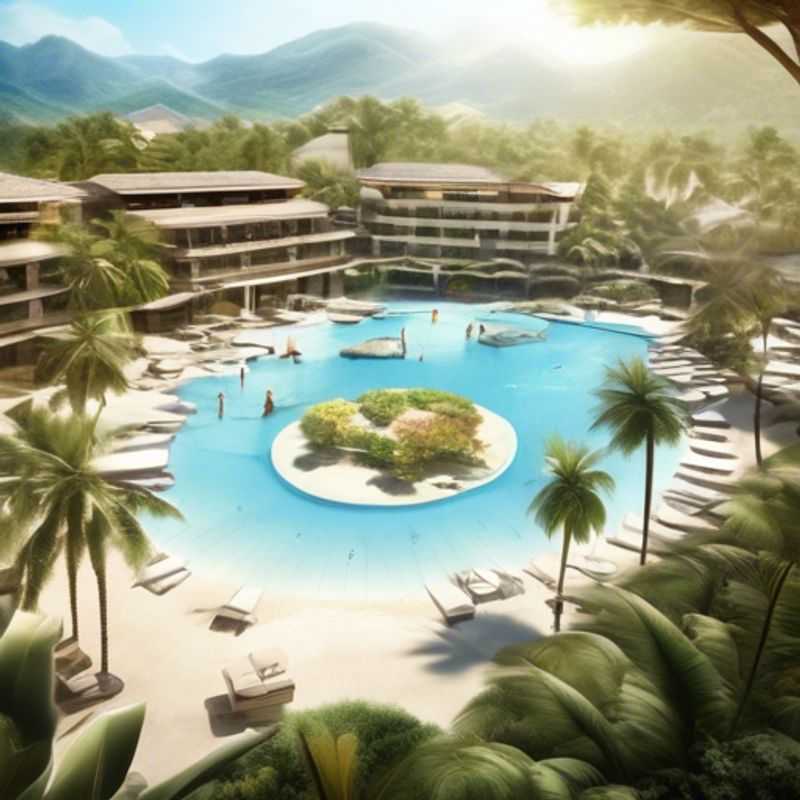
Keeping Your AI Model Sharp: Continuously Monitoring Outputs and Refining Prompts
Continuously monitoring model outputs is crucial for ensuring the quality and relevance of AI-generated content over time. By regularly assessing the performance of the model, users can identify areas for improvement and make necessary adjustments to prompts. This iterative process helps maintain high standards and aligns the model outputs with user expectations.
Refining prompts involves tweaking the input queries to enhance the model's understanding and responsiveness. This can include rephrasing questions, providing additional context, or specifying desired formats. Regularly updating prompts based on feedback and output analysis ensures that the model remains effective and accurate.
When estimating a plan for this process, consider the following potential costs:
- Software subscriptions for advanced monitoring tools that analyze outputs and provide insights.
- Consultation fees for experts who can help refine prompts and optimize model performance.
- Training costs for teams to effectively utilize monitoring tools and understand output evaluation.
By investing in these areas, organizations can significantly enhance their model's output quality, ensuring it remains a valuable tool in their workflow.
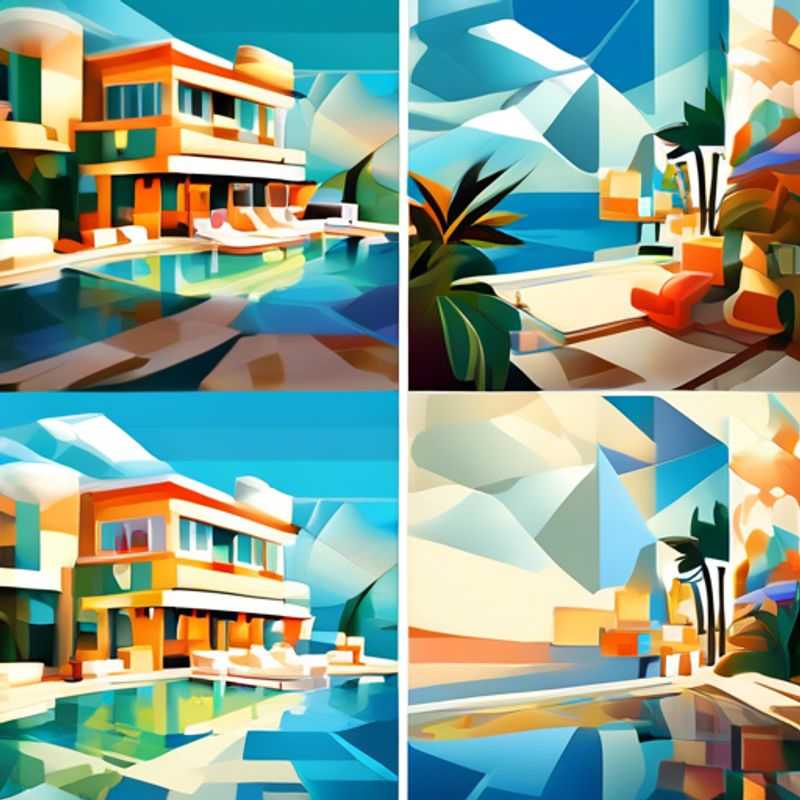